Training outpaces inference in AI infrastructure spending: Bernstein
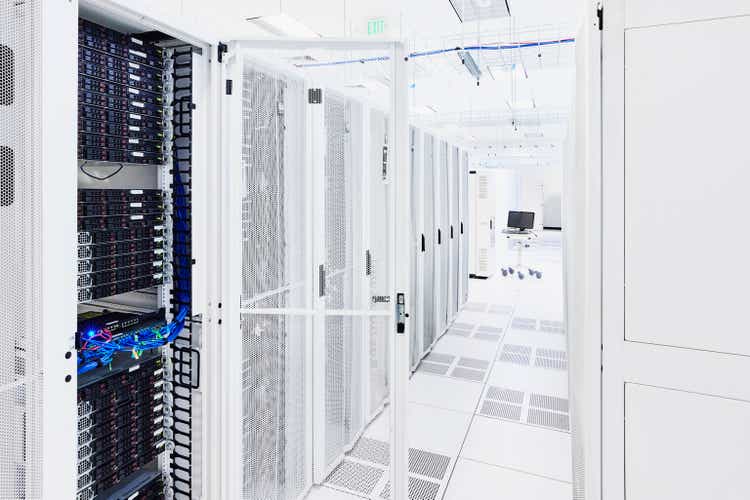
Thomas Barwick
As artificial intelligence companies clamor to build ever-growing large language models, AI infrastructure spending by Microsoft (NASDAQ:MSFT), Amazon Web Services (NASDAQ:AMZN), Google (NASDAQ:GOOG)(NASDAQ:GOOGL) and Meta Platforms (NASDAQ:META) is expected to reach a combined total of $160B in 2024, according to Bernstein Société Générale Group.
While investors generally believed more spending was going to inferencing, ever-increasing efficiencies in that area have prompted Bernstein to contend that training cost significantly more. Their latest data shows inferencing only accounts for about 5% of AI infrastructure spending.
Bernstein finds that every new LLM requires about 10 times in infrastructure costs over the previous model. For example, OpenAI’s GPT-2 was trained on a cluster of chips costing about $3M, while GPT-3 required about $30M worth of hardware to train. GPT-4 was then trained on 25,000 A100s, which cost about $300M.
Using this history as guidance, Bernstein determines GPT-5 will require 100,000 of Nvidia’s H100s, which would cost about $3B, to train the model. To put this in perspective, OpenAI is projected to earn $3.7B in sales in 2024.
“For Dell (NYSE:DELL) and Hewlett Packard Enterprise (NYSE:HPE), we believe that their AI fortunes will remain tethered to training and Tier 2 hyperscalers for the foreseeable future, where profits are very low, as opposed to on-premise inferencing, where margins are likely to be much higher,” said Bernstein analysts, led by Toni Sacconaghi, in a Wednesday investor note.
“Potential for continued strong Gen-AI training dynamics is likely constructive for NVIDIA (NASDAQ:NVDA) at this point, though questions around the pace and trajectory of Gen-AI inference, however, may come more at the expense of their peers who have broadly acknowledged NVIDIA’s training dominance and have hence focused on inference as the bulk of their long-term opportunity,” he added.
Leading LLM builders are already pre-buying Nvidia’s Blackwell to prepare for next-generation models.
However, Bernstein warns “there are many precedents for tech companies over-building capacity and then experiencing a major digestion cycle, including within the server market specifically and the telco/fiber market.”
“In particular, we worry about a scenario where a new model generation disappoints, in turn leading to a deceleration in training spend, but inferencing adoption does not ramp steeply enough to ‘pick up the slack,’ creating a major air pocket in spend,” Bernstein added.