AI chip spend must rise to meet even ‘modest’ expectations for LLM developers: Barclays
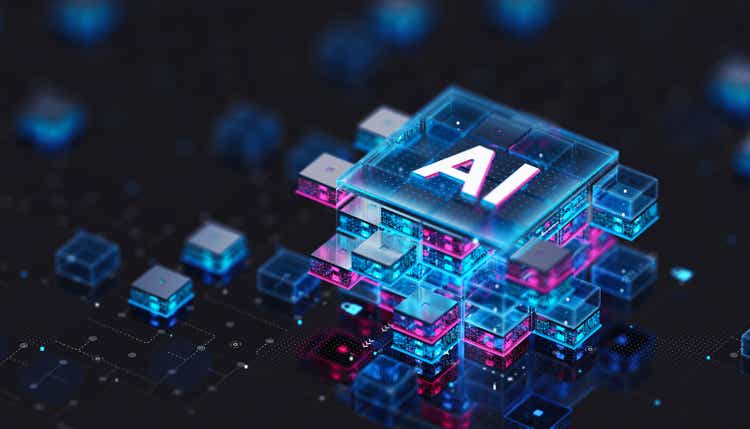
BlackJack3D
Barclays said that AI chip spend must increase to meet even the modest expectations for model developers, while highlighting important takeaways for the semiconductor space.
Analyst Tom O’Malley said the sell-off of AI chip names since Nvidia’s (NVDA) earnings has reverberated through the entire AI ecosystem, allowi9+g the question of “are we approaching the peak” to grow louder. The analyst believes this view does not properly factor in the compute needs of tomorrow.
The analyst added that they can count at least 9 individual organizations developing leading-edge, large parameter count large language models, or LLMs. There is no promise that the majority of these companies will continue to push onto the next iteration of model development for several reasons (return on investment, or ROI, funding, training data limits, and roadmap issues, among other things). But, only few companies are pushing through, for just several more model iterations demands an incredibly large amount of compute.
This demand is well in excess of the industry’s current expectations, and the analyst identifies three factors below as key takeaways for their semis coverage.
The first being, is the need to ease into projected capacity. The analyst said they estimate that the required compute resources needed to power only three frontier models of about 50T parameters each would require nearly 20M chips for training alone by 2027. One main reason for the large unit demand is that new model compute demand is expected to grow at a significantly faster rate than the rate they see today and faster than they are expecting accelerator performance to scale.
Secondly, according to the analyst, there is a way for Merchant and Custom to both win. They believe in a two-pronged approach when it comes to AI accelerators, with merchant solutions being more apt for training and inferencing frontier models (mainly Nvidia, but also AMD (AMD) and accelerator startups), while hyperscale custom silicon would be used for more specialized workloads within the data center of the chip’s developer.
The analysts added that they have seen this generally play out as expected, with limited exceptions (such as Apple using TPU), and they continue to expect this market bifurcation to play out moving forward.
The third being, that robust markets will exist for inference. O’Malley noted that Nvidia’s recent claims that about 40% of its chips are being used for inference, combined with other accelerator providers’ renewed focus on the inference market, underpins an emerging portion of the AI compute equation.
Inference will be the main conduit for monetizing the frontier models which are currently in development. Provisioning more resources to this side of the compute equation in order to drive down inference token costs will lead to greater ROI of models in development, according to the analysts. Lastly, the recent introduction of OpenAI’s o1 model is widely believed to signal promise from incremental, compute resources committed to inference steps, the analyst added.