AMD Downgrade: Don’t Make The Mistake Of Buying Just Because It’s Cheap
Summary:
- Many analysts are recommending to buy Advanced Micro Devices, citing its cheap valuation. Although it is important to properly understand why the stock is down 35% in the first place.
- The bullish narrative last year was that while Nvidia Corporation has dominated the AI training market, AMD could seize market share in the inferencing market.
- We discuss just how excruciatingly difficult it has become for AMD to gain market share given Nvidia’s savvily clever strategies to keep customers entangled within its ecosystem during inferencing phase.
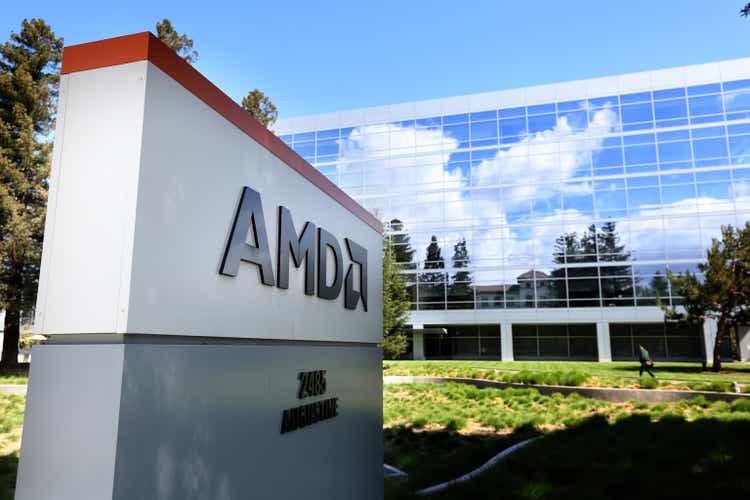
Justin Sullivan
Advanced Micro Devices (NASDAQ:AMD) stock has fallen by around 8% year-to-date, and is on track to end the year down in the single-digit percentages. AMD was actually rallying strongly at the beginning of 2024, and the share price peaked at around $211 in March 2024 before shedding about 35% of its value, deep into bear market territory.
In the previous article, we had discussed the growth opportunities around AI PCs over the coming years, although the initial adoption rate among end-consumers has been rather slow. And in the preceding article, we had covered how AMD could have a chance to catch up and gain market share amid companies sticking to traditional, simpler AI workloads given the imperfections of generative AI.
However, with the AI revolution now evolving towards the age of “agents”, AMD is falling concerningly behind its tech peers. The bull case narrative earlier this year was that while Nvidia (NVDA) has dominated the AI training market, AMD could seize market share in the inferencing market. But this is not playing out as expected. AMD stock is being downgraded to a ‘hold’ rating.
Bear case
On the Q3 2024 AMD earnings call, CEO Lisa Su raised the Data Center GPU revenue projection for this year to over $5 billion (previously $4.5 billion). Although this was still underwhelming for the bulls that were expecting much greater market share capture by now.
Many analysts have been recommending to buy AMD stock lately citing its cheap valuation. Although before one decides to invest, it is important to understand why the bullish narrative around “inferencing” hasn’t played out so far, as well as the growth prospects over the coming years.
In an article earlier this year, we had delved into how Nvidia is strongly positioned to sustain dominant market share as we shift from the training to the inferencing phase, given the efficacy of its HGX supercomputers (bundling four to eight GPUs together).
HGX systems come with an optimized software environment to run deep learning frameworks like TensorFlow and PyTorch.
… these deep learning frameworks like TensorFlow and PyTorch offer optimized libraries for both training and inference on H100s and Nvidia HGX systems. These libraries ensure smooth transitions between the training and inferencing stages.
If customers indeed decide to leverage these software libraries that strive to facilitate seamless transitions from training to inferencing on the same chips and servers, it would undermine the bearish argument that the data center customers will purchase massive numbers of new chips from alternative providers to run their inferencing workloads.
Lo and behold, this is exactly what has been playing out over the last several months, to AMD’s detriment.
we’re seeing inference really starting to scale out for our company. We are the largest inference platform in the world today because our installed base is so large and everything that was trained on Amperes and Hoppers inference incredibly on Amperes and Hoppers.
And as we move to Blackwells for training foundation models, it leads behind it a large installed base of extraordinary infrastructure for inference. And so we’re seeing inference demand go up.
– CEO Jensen Huang, Nvidia Q3 FY2025 earnings call (emphasis added)
Essentially, once customers are done with training their AI models using Nvidia’s mighty chips, these GPUs are then being deployed for inferencing workloads, subduing the need to purchase an entirely new batch of silicon from AMD. And this trend is only likely to continue over the next few years as data centers upgrade their infrastructure with the most powerful chips available from Nvidia for new model training workloads. So when Nvidia launches “Rubin” new year, the current shipment of Blackwell chips will subsequently be used for inferencing.
This is the reason AMD’s stock is down 35% from its peak, and why its GPU sales numbers are so underwhelming. Looking forward, AMD’s AI chip sales may continue to be unexciting given Nvidia’ clever strategies to continuously augment the value proposition of its hardware via savvy software advancements.
when you’re training new models you want your best new gear to be used for training right which leaves behind gear that you used yesterday well that gear is perfect for inference and so there’s a trail of free gear there’s a trail of free infrastructure behind the new Infrastructure that’s Cuda compatible and so we’re very disciplined about making sure that we’re compatible throughout.
We also put a lot of energy into continuously reinventing new algorithms so that when the time comes the hopper architecture is two, three, four times better than when they bought it so that infrastructure continues to be really effective – CEO Jensen Huang on BG2 Podcast (emphasis added)
Nvidia’s extensively thought-out strategies, including cohesive CUDA compatibility across GPU generations and innovation of new algorithms to extend the use cases of its chips, keeps customers entangled into the ecosystem as workloads shift from training to inferencing. Hence, the company is well- positioned to sustain market dominance throughout the inference phase, narrowing the window for AMD’s technology to gain market prominence.
Furthermore, while AMD is still striving to catch up on the software front with its open-source ROCm platform to challenge CUDA, Nvidia is building upon its leadership position by providing inferencing solutions for agentic workloads, namely its NIM and NeMo offerings
NVIDIA AI Enterprise, which includes NVIDIA NeMo and NIM microservices is an operating platform of agentic AI. Industry leaders are using NVIDIA AI to build Co-Pilots and agents. Working with NVIDIA, Cadence, Cloudera, Cohesity, NetApp, Nutanix, Salesforce, SAP and ServiceNow are racing to accelerate development of these applications with the potential for billions of agents to be deployed in the coming years.
…
Nearly 1,000 companies are using NVIDIA NIM and the speed of its uptake is evident in NVIDIA AI Enterprise monetization. We expect NVIDIA AI Enterprise full year revenue to increase over 2 times from last year and our pipeline continues to build.
– CEO Jensen Huang, Nvidia Q3 FY2025 earnings call
Meanwhile, AMD’s executives have not mentioned the words ‘agent’ or ‘agentic’ even once on their earnings calls, and are yet to introduce GPUs and accompanying software that are powerful enough to process such complex workloads. The extensive lead that Nvidia continues to possess over AMD certainly makes it increasingly difficult for Lisa Su’s company to seize a larger slice of market share.
Bull case
In line with previous historical tech cycles, the rise of open-source technology is always welcome as it accelerates adoption. In this generative AI revolution, Meta Platforms (META) has been playing a key role in making the technology universally accessible by open-sourcing its Llama AI models.
Realizing that Meta’s open-source models are well-positioned to become ubiquitously used across industries due to the transparent code and cost advantages, the top chipmakers have been actively synchronizing with Llama advancements.
you’re seeing folks like NVIDIA and AMD and optimize their chips more to run Llama specifically well” – CEO Mark Zuckerburg, Meta Platforms Q3 2024 earnings call
This is a very smart move as it counters the growing risk of cloud providers designing their own chips, which are likely to be optimized for running their in-house AI models (e.g. Google TPUs are optimized for running Gemini). The growing popularity of Meta’s open-source models could sustain demand for AMD and Nvidia’s silicon within cloud environments, particularly as they become better suited for processing Llama-based workloads.
Moreover, AMD in particular has been making significant strides in encouraging Meta to deploy its chips for various internal workloads, including both CPUs and GPUs.
In cloud, EPYC CPUs are deployed at scale to power many of the most important services, including Office 365, Facebook, Teams, Salesforce, SAP, Zoom, Uber, Netflix and many more. Meta alone has deployed more than 1.5 million EPYC CPUs across their global data center fleet to power their social media platforms.
…
Meta announced they have optimized and broadly deployed MI300X to power their inferencing infrastructure at scale, including using MI300X exclusively to serve all live traffic for the most demanding Llama 405B frontier model. We are also working closely with Meta to expand their Instinct deployments to other workloads where MI300X offers TCO advantages, including training.
– CEO Lisa Su, AMD Q3 2024 earnings call (emphasis added)
So as AMD’s chips become increasingly optimized for running Meta’s Llama series, it could indeed open the doors to greater customer deployments across industries, particularly as it offers a more cost-effective alternative to Nvidia’s technology.
Additionally, as CEO Lisa Su mentioned on the call, the possibility of AMD’s chips being used for training some of Meta’s models could further augment the value proposition of its GPUs, helping counter Nvidia’s strong hold of the AI training market to some extent.
Additionally, consider the fact that Nvidia’s strong pricing power also benefits AMD in a way. While the underdog has obviously been pricing its GPUs below that of Nvidia’s silicon, AMD has still been able to charge sufficiently high prices to cover its rising R&D costs and deliver healthy profit margin expansion, as Nvidia has left ample room for AMD to charge high prices as well.
Data Center segment operating income was $1 billion or 29% of revenue compared to $306 million or 19% a year ago. Data Center segment operating income more than tripled compared to the prior year, driven by higher revenue and operating leverage.
Broadly across the company, AMD’s operating margin was 25% in Q3 2024, marking an expansion from the 22% margin both in the preceding sequential quarter, as well as the prior year’s Q3 2023 quarter.
Often times when a company is trying to catch up to a competitor with such a wide lead, it incurs aggressively low pricing strategies coupled with mounting R&D costs, which ends up hurting profit margins, even leading to losses in many cases. Conversely, AMD seems to be in a relatively better position to catch up to Nvidia while still delivering profit margin expansion for shareholders.
The investment case for AMD
AMD’s healthy profit margins indeed are flowing down to the bottom line, driving EPS growth for shareholders. Relative to some competitors and cloud provider stocks, AMD offers the highest projected EPS growth rate over the next 3-5 years, at 42.24%.

Nexus Research, data compiled from Seeking Alpha
Expected EPS growth rates are key to comprehensively valuing a stock. While investors commonly use the Forward PE ratio to determine a stock’s valuation, the Forward Price-Earnings-Growth (Forward PEG) is a more thorough valuation metric, as it adjusts the Forward PE ratio by the anticipated EPS growth rates.
AMD currently trades at over 40x forward earnings (non-GAAP), which can appear expensive. However, adjusting AMD’s and competitors’ Forward PE ratios by their projected EPS growth rates gives us the following Forward PEG multiples.

Nexus Research, data compiled from Seeking Alpha
For context, a Forward PEG multiple of 1x would implicate that the stock is trading around its fair value.
Acknowledging this, not only is AMD the cheapest out of the AI stocks, but it is also trading below its fair value.
Though the cheap valuation is indeed indicative of the market’s deep concerns around AMD’s ability to gain market share, particularly as Nvidia moves onto offering powerful technology that can process “agentic” workloads. The trend of Nvidia’s training GPUs being subsequently used for inferencing could be a difficult cycle to break for AMD, in turn subduing its data center revenue growth prospects over the coming years.
With Nvidia trading at a relatively cheap valuation as well at 1.20x Forward PEG, it certainly undermines the appeal of buying AMD over the market leader.
That being said, I wouldn’t recommend selling AMD either based on these concerns, particularly for investors that have been invested in the stock for many years with a low price-entry point. In which case holding onto the stock could still be worthwhile in the event that the company’s technology gradually gains prevalence amid price-performance improvements for processing open-source Llama-based workloads, or even less compute-intensive workloads like fine-tuning pre-trained models.
But for investors looking to put new capital to work in AI stocks, AMD is an unexciting option despite the declining valuation, and would recommend NVDA instead.
AMD stock has been downgraded to a ‘hold’.
Analyst’s Disclosure: I/we have a beneficial long position in the shares of NVDA either through stock ownership, options, or other derivatives. I wrote this article myself, and it expresses my own opinions. I am not receiving compensation for it (other than from Seeking Alpha). I have no business relationship with any company whose stock is mentioned in this article.
Seeking Alpha’s Disclosure: Past performance is no guarantee of future results. No recommendation or advice is being given as to whether any investment is suitable for a particular investor. Any views or opinions expressed above may not reflect those of Seeking Alpha as a whole. Seeking Alpha is not a licensed securities dealer, broker or US investment adviser or investment bank. Our analysts are third party authors that include both professional investors and individual investors who may not be licensed or certified by any institute or regulatory body.