Nvidia Upgrade: Fortune Favors Patience
Summary:
- Various investors will have bought Nvidia Corporation stock at different stages of the phenomenal rally over the past two years, hoping they are not too late to profit from the AI.
- The market is increasingly concerned over whether Nvidia’s customers will see justifiable returns on investments, given the underwhelming monetization trends and the struggle of AI start-ups, creating risks for NVDA.
- However, shareholders should not make the mistake of selling the stock based on these concerns, given the latest industry findings and analogous research.
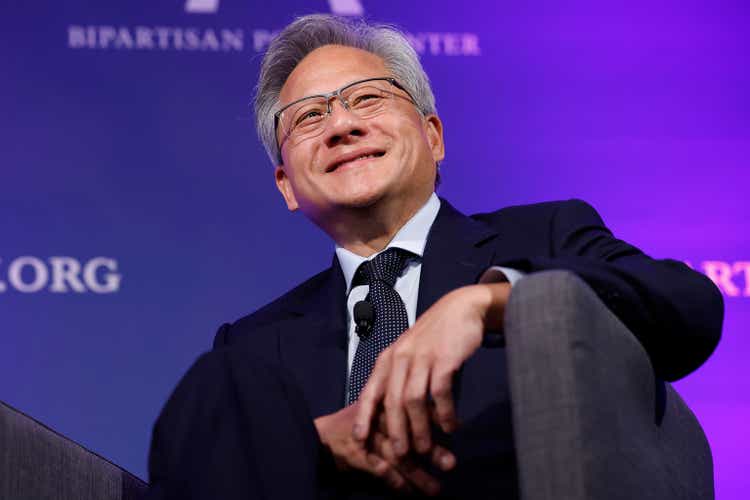
Chip Somodevilla
After an incredible rally in 2023, Nvidia Corporation (NASDAQ:NVDA) has rallied by another 186% so far this year. Various investors will have bought Nvidia stock at different stages of the phenomenal rally over the past two years, hoping they are not too late to profit from the AI revolution.
In our previous article, we extensively discussed Nvidia’s growing revenue opportunities around the robotics revolution. We covered the hardware revenue prospects via Nvidia’s Jetson supercomputers, as well as the promising, recurring software revenue potential around “NVIDIA Omniverse Enterprise,” which is $4,500 per GPU on an annual basis. Essentially, this growing market extends the bull case for NVDA way beyond Large Language Models (LLMs) and chatbots.
However, regarding the current growth narrative around generative AI, the market is increasingly concerned over whether Nvidia’s customers will see justifiable returns on investments. This is given the underwhelming monetization trends among some big tech companies, and the struggle of AI start-ups to generate returns for venture capital investors.
Nonetheless, evidence of GenAI investments starting to pay off is now arising, and historical tech analogies implicate that it would be foolish to sell NVDA based on return-on-investment (ROI) concerns from skeptics. Nvidia stock is being upgraded to a “buy” rating.
The ROI of generative AI
The largest tech companies have been investing billions of dollars into Nvidia’s GPUs. They are building up new-age infrastructure for facilitating generative AI, either for supporting first-party workloads, or in the case of cloud service providers, renting out computing systems to third-party companies and start-ups.
However, large-cap tech companies are still struggling to prove to investors that the GenAI investments they are making can generate worthwhile returns. For example, Microsoft (MSFT) has yet to disclose the adoption and revenue run rates of its Copilot assistant. Investors will be closely watching Salesforce’s (CRM) Q3 earnings report next week (expected December 3rd) to gauge the growth prospects around its “Agentforce” service powered by Nvidia’s technology.
And then we have the “start-ups” layer of the tech industry, whereby founders are striving to build natively AI companies from the ground up to revolutionize software in this new era.
Creating the large-language models that power generative AI often requires hundreds of millions of dollars in upfront investment before returning a cent of revenue. Many startups are discovering they don’t have the resources and runway to get there.
There were a lot of companies that raised on a big vision, but not tangible examples and actual detail,” said Shaun Johnson, a founding partner at the AI-focused venture firm AIX Ventures.
If venture capitalists continue to see insufficient returns on their investments, they could pull back on funding rounds to support new start-ups, subsequently weakening end-demand for Nvidia’s hardware and software.
Moreover, data analytics firm “Databricks,” which recently forged a partnership with AWS to enable its clients to use Amazon’s custom chips in pursuit of better price-performance, also raised concerns around the monetization prospects of generative AI.
Naveen Rao, vice president of generative AI at cloud data firm Databricks, said that some 90% of generative AI experiments aren’t making it beyond the lab. “Accuracy and reliability is a big problem,” he said.
There is an argument to be made that just because GenAI cannot produce completely accurate and reliable results today does not mean investments in the space is going to stop. Conversely, tech firms will strive to come up with new training/ inferencing methods to improve the accuracy of AI models and applications, potentially conducive to greater demand for compute power from Nvidia, depending on the approaches used.
Furthermore, Google (GOOG)(GOOGL) recently released a report after having surveyed 2,500 enterprises on their generative AI deployment journeys, and uncovered how most companies are already seeing tangible results.
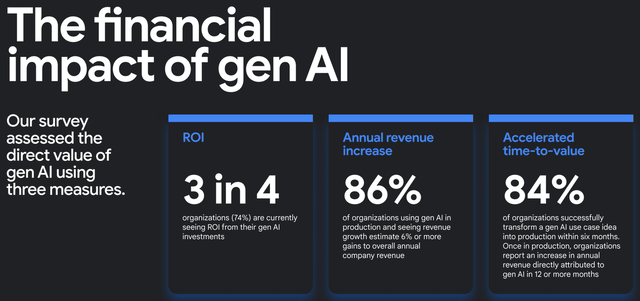
Note: number of companies that answered each survey question can vary, and is less than 2500 in each case (Google)
As per Google’s “The ROI of GenAI” report, 74% of surveyed organizations are witnessing ROI on their generative AI investments. And it’s not just limited to productivity improvements, but also top-line revenue growth advancements.
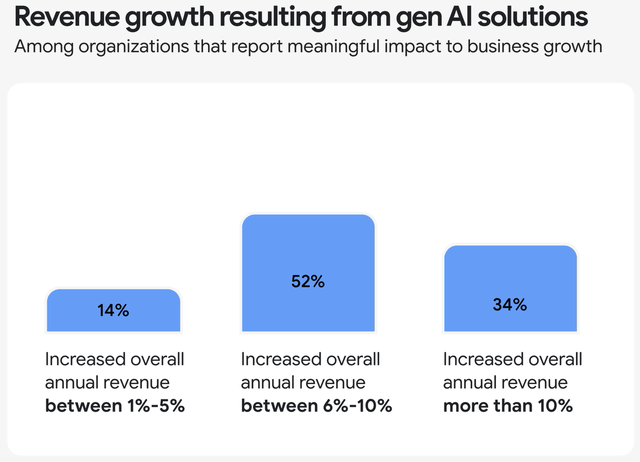
So while the bears’ continue to doubt the ROI prospects from heavy GenAI investments, evidence of tangible gains is already arising, in favor of Nvidia and the largest cloud providers.
Skeptics still question whether the returns will be commensurate with the level of capital that the tech giants continue to pour into this new technology. Nvidia’s GPUs are particularly expensive, raising the bar for ROI requirements.
Additionally, the bears also point to the fact that since the launch of ChatGPT two years ago, there have no other “killer AI applications” that justify the large-scale capital expenditures by big tech.
Nonetheless, as per historical technology cycles, the cost of computing should eventually decline to propel greater feasibility and investment return profiles.
AI technology is undoubtedly expensive today. And the human brain is 10,000x more effective per unit of power in performing cognitive tasks vs. generative AI. But the technology’s cost equation will change, just as it always has in the past. In 1997, a Sun Microsystems server cost $64,000. Within three years, the server’s capabilities could be replicated with a combination of x86 chips, Linux technology, and MySQL scalable databases for 1/50th of the cost. And the scaling of x86 chips coupled with open-source Linux, databases, and development tools led to the mainstreaming of AWS infrastructure. This, in turn, made it possible and affordable to write thousands of software applications, such as Salesforce, ServiceNow, Intuit, Adobe, Workday, etc. These applications, initially somewhat limited in scale, ultimately evolved to support a few hundred million end-users, not to mention the impressive scaling of Microsoft Azure that supported ubiquitous applications such as Office 365. Over the last decade, these applications have evolved and helped create hundreds of billions of dollars in shareholder value, providing even more evidence for the cliché that people tend to overestimate a technology’s short-term effects and underestimate its long-term effects. Nobody today can say what killer applications will emerge from AI technology. But we should be open to the very real possibility that AI’s cost equation will change, leading to the development of applications that we can’t yet imagine.
– Kash Rangan, Senior Equity Research Analysts at Goldman Sachs (emphasis added).
For context, when Amazon initially began investing heavily in establishing AWS during the early and mid-2000s, Wall Street analysts were skeptical of any payoffs from these investments, failing to recognize how cloud computing could replace traditional, on-premises computing systems. In hindsight, AWS turned out to be an absolute revenue-juggernaut, with cloud computing becoming the basis for the popular Software-as-a-Service (SaaS) applications we know today.
Similarly, Nvidia has its history of disproving skeptics, particularly around its now-envied CUDA software platform for programming chips for any use case the developer wants.
For ten years, Wall Street asked Nvidia, “why are you making this investment? No one’s using it.” And they valued it at $0 in our market cap. And it wasn’t until around 2016, ten years after CUDA came out, that all of a sudden people understood this is a dramatically different way of writing computer programs and it has transformational speedups that then yield breakthrough results in artificial intelligence.
– Bryan Catanzaro, VP of Applied Deep Learning at Nvidia.
So for the bears that point to the absence of “killer AI applications” and lack of visibility thereof, keep in mind that years after its launch, CUDA ended up solving problems that Nvidia’s own executives and developers hadn’t imagined themselves. This is essentially the beauty of inventing an entirely new form of computing. Patience is a virtue.
Moreover, Nvidia is already making notable progress on improving the price-performance of its GPUs to advance ROI prospects for its customers.
Our large installed base and rich software ecosystem encourage developers to optimize for NVIDIA and deliver continued performance and TCL improvements. Rapid advancements in NVIDIA’s software algorithms boosted Hopper inference throughput by an incredible 5 times in one year and cut time to first token by 5 times. Our upcoming release of NVIDIA NIM will boost Hopper Inference performance by an additional 2.4 times. – CEO Jensen Huang, Q3 2025 Nvidia earnings call (emphasis added).
On a broader industry-wide level, the cost of inference compute is falling rapidly thanks to the rise of open-source AI models.
In fact, the price decline in LLMs is even faster than that of compute cost during the PC revolution or bandwidth during the dotcom boom: For an LLM of equivalent performance, the cost is decreasing by 10x every year. Given the early stage of the industry, the time scale may still change. But the new use cases that open up from these lower price points indicate that the AI revolution will continue to yield major advances for quite a while.
…
When GPT-3 became publicly accessible in November 2021, it was the only model that was able to achieve an MMLU of 42 — at a cost of $60 per million tokens. As of the time of writing, the cheapest model to achieve the same score was Llama 3.2 3B, from model-as-a-service provider Together.ai, at $0.06 per million tokens. The cost of LLM inference has dropped by a factor of 1,000 in 3 years. – Venture capital giant Andreessen Horowitz (emphasis added).
The continuous decline in computing costs improves the accessibility of the technology for developers and companies, which should spur innovations cultivating in “killer AI applications.” Additionally, it also enables enterprises to offer new-age products and services at more affordable prices to end-customers, ultimately paving the way to ubiquitous generative AI usage.
Risks to the bull case
While industry-wide efforts to bring down the cost of computing and encourage more wide-spread adoption of AI should be advantageous for Nvidia, one can’t ignore the endeavors against Nvidia as the tech industry strives to make generative AI more affordable.
In a previous article, we covered how the UXL Foundation is striving to build an open-source alternative to Nvidia’s CUDA platform, essentially easing the migration process between any chip, and helping enterprise customers avoid lock-in. This tech consortium is being led by some leading semiconductor and software companies, including Arm Holdings (ARM) and Google Cloud.
That being said, it remains to be seen whether these consortiums will actually be successful at challenging Nvidia’s moat. Though, it is still important to acknowledge the collective efforts being made by some of Nvidia’s key customers and suppliers to dethrone the company from its “king of AI” stature.
Furthermore, cloud providers are also making notable progress in challenging Nvidia’s chip superiority. They are designing their own silicon in such a manner that it deeply integrates with their cloud servers and broader data center systems, yielding performance advantages and cost efficiencies that can be passed onto customers.
In fact, on Amazon’s Q3 2024 earnings call, CEO Andy Jassy proclaimed just how high the demand is for their custom silicon (emphasis added):
while we have a deep partnership with NVIDIA, we’ve also heard from customers that they want better price performance on their AI workloads. As customers approach higher scale in their implementations, they realize quickly that AI can get costly. It’s why we’ve invested in our own custom silicon in Trainium for training and Inferentia for inference. The second version of Trainium, Trainium2 is starting to ramp up in the next few weeks and will be very compelling for customers on price performance. We’re seeing significant interest in these chips, and we’ve gone back to our manufacturing partners multiple times to produce much more than we’d originally planned.
AWS is clearly making headway in inducing greater adoption of its in-house chips, including by striking partnerships with AI-centric companies like Databricks and Anthropic.
And then we have Google Cloud, which remains in the lead when it comes to custom chips for AI workloads, offering its Tensor Processing Units (TPUs) since 2018. In fact, in a recent article we discussed how Google Cloud is the only hyperscaler gaining market share in 2024, and how it is “running circles around Microsoft Azure” on the “custom chips” front thanks to being less reliant on Nvidia’s GPUs.
Additionally, AI start-ups like Groq and Cerebras are also specifically designing chips to handle AI inferencing computations, offering cheaper alternatives over Nvidia’s GPUs.
The point is, the broader tech industry’s efforts to continuously improve the price-performance of generative AI computations and make the technology universally accessible may indeed incur alternatives to Nvidia’s platform that is likely to eat into its market share.
The investment case for Nvidia stock
NVDA currently trades at around 48x forward earnings. Although it is imperative to also consider the rate at which a company is growing its earnings, not just what the earnings will be, when assessing a stock’s valuation.
Despite rising competition, Nvidia’s pricing power remains second to none, conducive to enviable profit margins. Gross margins continue to hover between 70-75%, while the net margins remain above 50%, which means the majority of the revenue generated by the company flows down to the bottom line, boosting EPS for shareholders.
Unsurprisingly, out of all the Magnificent 6 stocks, NVDA has the highest projected EPS growth rate at 37.53%.
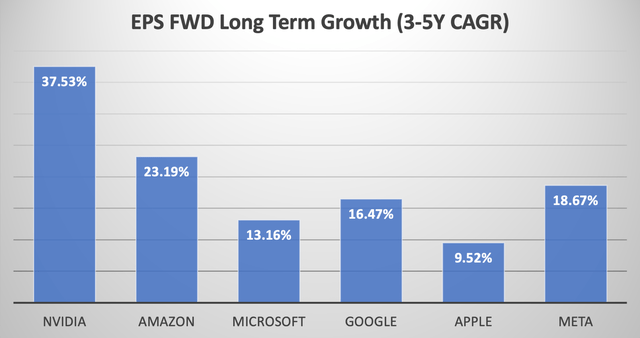
Nexus Research, data compiled from Seeking Alpha
Note that Tesla (TSLA) has been excluded from the group of ‘AI Winners’ usually dubbed as “Magnificent 7,” given the stock’s detachment from fundamentals lately.
Now, when we adjust each stock’s Forward P/E ratio by the expected EPS growth rates, we obtain the following Forward Price-Earnings-Growth (Forward PEG) multiples.
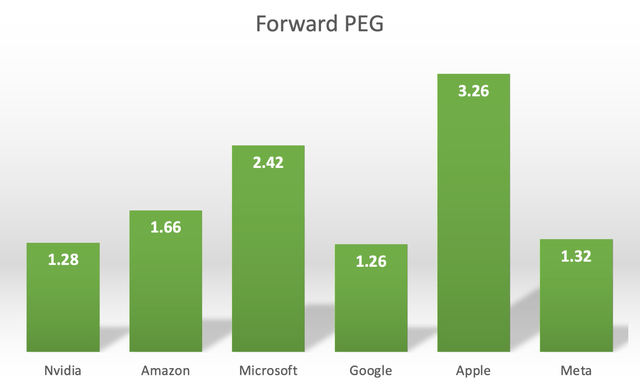
Nexus Research, data compiled from Seeking Alpha
Barring GOOG whose valuation is currently being discounted amid its antitrust challenges, NVDA remains one of the cheapest AI stocks.
So at this valuation, Nvidia’s shares are worth buying. Although a note of caution here alongside the rating upgrade. NVDA remains a volatile stock prone to significant draw-downs in the share price. So investors that have been on the sidelines considering whether to purchase the stock should only invest if they are ready to buy more on any pullbacks, keeping the long-term growth prospects in mind.
At present, Nvidia remains the king of AI.
Analyst’s Disclosure: I/we have a beneficial long position in the shares of NVDA either through stock ownership, options, or other derivatives. I wrote this article myself, and it expresses my own opinions. I am not receiving compensation for it (other than from Seeking Alpha). I have no business relationship with any company whose stock is mentioned in this article.
Seeking Alpha’s Disclosure: Past performance is no guarantee of future results. No recommendation or advice is being given as to whether any investment is suitable for a particular investor. Any views or opinions expressed above may not reflect those of Seeking Alpha as a whole. Seeking Alpha is not a licensed securities dealer, broker or US investment adviser or investment bank. Our analysts are third party authors that include both professional investors and individual investors who may not be licensed or certified by any institute or regulatory body.