What To Watch For In Nvidia Earnings: The GPU Shortage And GH200
Summary:
- The AI bull rush in 2023 has greatly rewarded tech stock investors, particularly in the case of Nvidia Corporation.
- The GPU shortage is causing major players like Microsoft and Amazon to wait for more chip supply.
- Investors should watch for updates on customer allocation utilization, CoWoS packaging improvements, and the development of the GH200 GPU on the earnings call.
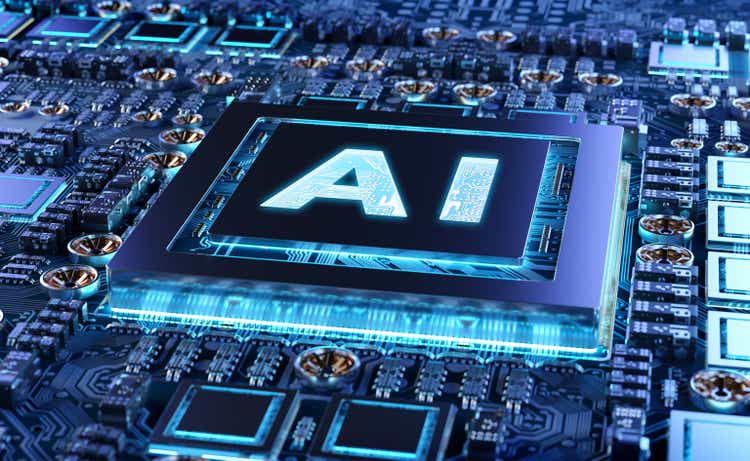
Guillaume/iStock via Getty Images
The AI bull rush throughout 2023 has greatly rewarded those that bought and held tech stocks through the 2022 downturn. In typical Wall Street fashion, they have become entranced with the potential that AI holds and how to make money off that. AI has captured the imagination of analysts, while the industry is facing a much different picture in reality. NVIDIA Corporation (NASDAQ:NVDA), is the AI-darling stock. A provider of highly capable GPUs, which are particularly useful for AI applications, Nvidia has seen a parabolic increase in demand and a similar parabolic movement in stock price. Demand increased significantly faster than supply could.
The GPU Shortage
The Cause
To understand the cause of this shortage, we must first delve a bit into the chip manufacturing process.
Nvidia’s demand for chips to fill its GPUs far exceeds the total production capacity of Taiwan Semiconductor Manufacturing Company (TSM). Without the ability to procure a sufficient amount of chips, Nvidia gives “allocations” to major players (Microsoft, Amazon, Google, etc.). When a customer is “at capacity” it means they’ve purchased their entire allocation and will have to wait for more supply.
What to watch for #1 – Who’s at capacity?
One thing to monitor on the earnings call is any reference to customers being “at capacity.” Functionally, this means that that specific customer: 1) won’t get any more AI-specialized chips until Nvidia provisions more allocations; and 2) is out-innovating its supply of chips, and is currently limited by computing power, not pace of innovation. Companies at capacity with Nvidia are likely on the leading edge of AI model development and building backlogs of model enhancements while waiting on more chip supply. It’s unclear whether Nvidia will be transparent with allocation amounts and allocation utilization per customer, but any mention of this should be monitored carefully.
The Cause, cont.
This shortage is caused by one specific step in the chip manufacturing process. Though photolithography tends to get a lot of attention in the fab process, there are a multitude of other extremely complicated and microscopic process steps. One such step is advanced packaging.
The use of HBM, high bandwidth memory, was created around 2015 and specialized in gaming applications, a market in which it struggled. To make HBM, chip designers vertically stacked a bunch of DRAM, dynamic random access memory, dies atop a ‘controller’ logic die. DRAM gives chips the ability to store a lot of memory without taking up space on the chip itself, allowing for accelerated performance. Both Advanced Micro Devices (AMD) and Nvidia’s leading AI GPUs (MI300X & H100, respectively) use HBM designs. As the volume of data flowing through data centers has rapidly grown, the need for massive increases in memory capacity has given HBM quite a compelling use case.
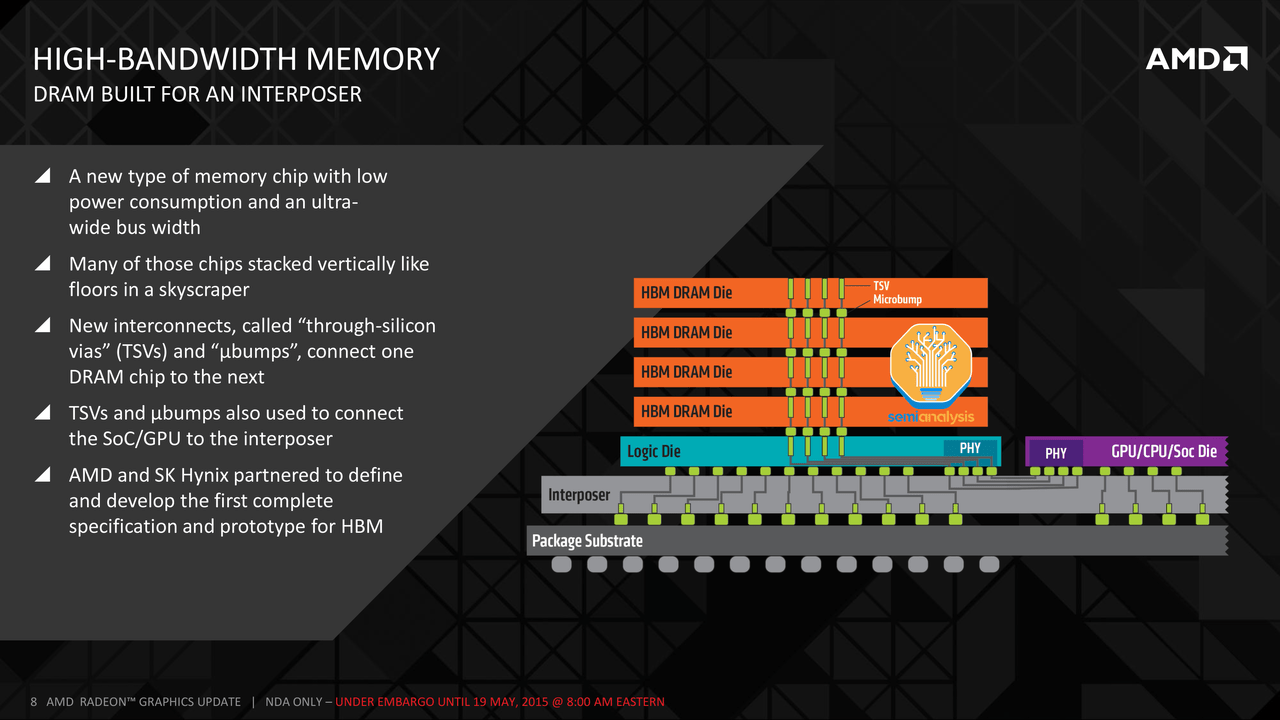
Semi Analysis, AI Capacity Constraints – CoWoS and HBM Supply Chain
Now with data centers hosting AI models of ever-growing parameter size, HBM solves a key bottleneck. AI model training and inference require the storage and retrieval of immense amounts of data from memory, this is known as the memory wall. Without sufficient memory space, AI models will not be able to continue growing. Nvidia’s H100 GPU uses only SK Hynix HBM3 designs, giving SK Hynix a commanding 95% market share.
HBM greatly increased manufacturing complexity as it necessitates the use of TSVs, through silicon vias, or tiny holes drilled through DRAM dies using photolithography to build vertical connections. HBM3E, a leading next-gen innovation, is expected to begin shipping in Q1 of 2024, so listen carefully for mention of that product.
Finally, we get to the true bottleneck: CoWoS advanced packaging. Chip-on-Wafer-on-Substrate architecture is a 2.5D advanced packaging design. CoWoS uses TSVs to connect various DRAM dies with each other, the logic die, and a silicon interposer. The interposer connects dies with the PCB, the circuit board that connects components and facilitates communication across the chip.
What to watch for #2 – Update on CoWoS capacity
This is colloquially known as ‘Flip-chip packaging’ and is currently bottlenecking the entire AI industry. Any mention of production capacity or related improvements for CoWoS packaging is important to investors.
Listen carefully for CoWoS-S updates. This is the packaging used for Nvidia data center GPUs A100, P100, and V100. Specifically, if CoWoS manufacturing has improved, the GPU shortage may resolve itself. If there has been little to no progress in CoWoS capacity, the shortage will persist.
Nvidia has stated they believe supply will increase in 2H 2023, so any mention of HBM, CoWoS, and any supply capacity warrants close listening.
The Effects
The effects of the GPU shortage have rippled across the AI industry and already created a difficult operating environment for AI startups. It’s easier for big names with big money to get H100 capacity allocations, but that hasn’t entirely excluded the startup ecosystem. Big tech companies have leaned more and more on in-house chip design in recent years, making them outright competitors to Nvidia. In other words, Nvidia’s largest customers are becoming key competitors. Although it’s not been formally stated, this likely impacts allocation decisions. While big tech is still a leading Nvidia customer, Nvidia may prefer to give more allocation to startups that aren’t competitive in chip design.
This is not true for all startups, though. Big cloud service providers and some noteworthy AI startups take up a vast majority of Nvidia’s allocations. This has cut off supply to many lower-tier competitors. They can neither get nor afford H100 GPUs, which can cost up to $380k per unit. Theoretically, this presents a tailwind for server OEMs like Super Micro Computer (SMCI), but SMCI is similarly impacted by allocation constraints. There simply aren’t enough Nvidia GPUs to go around. An H100 chip typically takes around 6 months to go from wafer to available-for-sale chip. The supply shortage has pervaded the entire AI ecosystem and will continue to hamper companies’ ability to innovate until it’s alleviated.
What to watch for #3 – GH200
Another key discussion on the earnings call will be the next-gen AI GPU the Nvidia GH200. The GH200 is not a new GPU, rather it’s a number of H100 GPUs connected with Grace Hopper CPUs, Nvidia’s new high-powered CPU. This is expected in production around roughly Q2 of 2024, so any updates on the development and production of that product will be important. This chip will serve as an intermediary between the H100 and the next-gen GPU, allowing for more compute power for customers and more pricing power for Nvidia. The GH200 is specialized for AI and is estimated to provide 3.5x more memory capacity and 3x more bandwidth than just an H100 GPU. These deliver more efficiency improvements for inference than they do for training, which is something Sam Altman, CEO of OpenAI, has said he prefers if forced to choose.
Conclusion
That rounds out this preliminary discussion on Nvidia earnings (expected post-market on August 23rd) and what I believe are the three most important things to listen for on the earnings call. This earnings release will be hugely impactful across tech and will cause ripple effects across the market. If there is good news about supply constraints, chip stocks could enter another phase of a bull run. However, if the supply situation is unchanged or has worsened, semis could be in for a protracted downturn.
Analyst’s Disclosure: I/we have a beneficial long position in the shares of SMCI either through stock ownership, options, or other derivatives. I wrote this article myself, and it expresses my own opinions. I am not receiving compensation for it (other than from Seeking Alpha). I have no business relationship with any company whose stock is mentioned in this article.
Seeking Alpha’s Disclosure: Past performance is no guarantee of future results. No recommendation or advice is being given as to whether any investment is suitable for a particular investor. Any views or opinions expressed above may not reflect those of Seeking Alpha as a whole. Seeking Alpha is not a licensed securities dealer, broker or US investment adviser or investment bank. Our analysts are third party authors that include both professional investors and individual investors who may not be licensed or certified by any institute or regulatory body.