Google: Why Is No One Talking About PaLM
Summary:
- There has been a lot of talk in recent weeks about whether OpenAI’s ChatGPT and the underlying GPT-3 language model (“LLMs”) poses a potential threat to Google Search’s future.
- Much of the conversation has been around whether Google’s LaMDA chatbot is capable of outperforming ChatGPT, or sufficient to dodge disruption.
- But a deeper dive into Google’s foray in LLMs would lead to the less talked-about Pathways AI Infrastructure, which powers its next-generation PaLM LLM that is 3x larger than GPT-3.
- The following analysis will provide an overview of both OpenAI and Google’s latest developments pertaining to LLMs, and gauge their implications on the tech giant’s longer-term prospects.
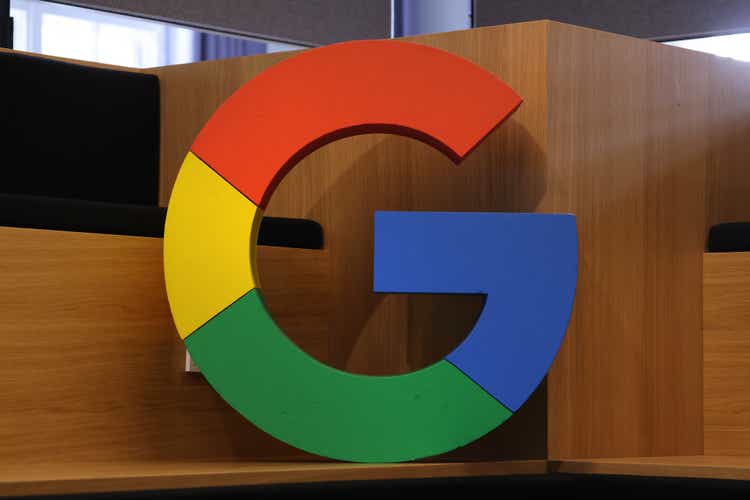
Sean Gallup
Given the sheer size of what Google (NASDAQ:GOOG) (NASDAQ:GOOGL) has grown into over the past decade – in terms of both its balance sheet and market share across various digital verticals spanning ads, video streaming, and cloud-computing – investors have gradually shifted focus from lucrative share gains to sustainability. Specifically, the market is fixed on how Google will continue to maintain its market leadership and sustain a long-term growth and profitability trajectory against disruption.
And OpenAI’s recent release of ChatGPT has only drawn up greater interest and attention on the sustainability of Google’s business model – particularly on Google Search and advertising, which is where the bread and butter is at. ChatGPT has provided the public with a glimpse into what large language models (“LLMs”) are capable of today. Admittedly, this has driven an outburst of speculation and analyses on whether Google’s market leadership in online search engines is at risk of looming disruption (we were one of them). And ironically, Google Search was likely the most-visited destination in the process of gathering relevant information (we’re guilty too).
Anyhow, we think OpenAI’s recent release of ChatGPT for public trial is a positive for Google. While the initial reaction might be that ChatGPT is very likely on track to replacing Google by providing much more accurate answers, not to mention a more convenient search process that would save through hours of scrolling through search results, it also draws traction and curiosity towards LLMs. More specifically, the recent attention over OpenAI’s ChatGPT has likely generated greater awareness into what Google has been doing in the field.
The following analysis will provide an overview of what Google has done in the realm of LLMs, how they compare to OpenAI’s GPT-3 (which currently powers ChatGPT), and walk through the key implications of said developments on Google’s core business – namely, Search ads. While acknowledging ChatGPT’s threat to Google Search is welcome, we believe the tech giant’s robust balance sheet, unwavering commitment to innovation, and sprawling market share remain key factors anchoring the sustainability of its longer-term growth trajectory.
Let’s Step Away from ChatGPT for a Minute
ChatGPT’s debut has not been all bad for Google. Yes, the chatbot has likely contributed to Google’s stock consistently underperforming against peers and the broader market in recent weeks, but it has also brought more interest and attention to what LLMs are, where the technology stands today, and more importantly, what Google has been doing about it. If anything, OpenAI’s recent decision to open ChatGPT to the public has likely put Google’s engineers on notice too to ensure the tech giant is nowhere close to falling behind.
From our recent series of coverage on both Microsoft (MSFT) and Twilio (TWLO), which analyze how OpenAI’s technologies could potentially impact their respective business models, we have observed from comments that much of investors’ focus is currently revolving around ChatGPT itself, rather than the underlying LLM – GPT-3 – that powers it. But it is important to acknowledge that the real threat is not the chatbot, but rather the verticals that GPT-3 and its successors stand to upend.
So what exactly are LLMs and GPT-3?
As previously discussed, language models in AI are transformers capable of learning from massive data sets to improve output over time:
One potential route addressing [limitations of NLP systems] is meta-learning, which in the context of language models means the model develops a broad set of skills and pattern recognition abilities at training time, and then uses those abilities at inference time to rapidly adapt to or recognize the desired task. In-context learning uses the text input of a pretrained language model as a form of tasks specification. The model is conditioned on a natural language instruction and/or a few demonstrations of the task and is then expected to complete further instances of the tasks simply by predicting what comes next.
Source: “Language Models are Few-Shot Learners”
And developments in this field have been evolving at a rapid pace, from Google’s “BERT” (Bidirectional Encoder Representations from Transformers) which we likely face daily without even noticing, to December’s spotlight feature, GPT-3, which powers ChatGPT.
GPT-3 is currently one of the largest language models available in the market, with 175 billion parameters. To better put into perspective GPT-3’s performance capabilities:
GPT-3 is more than 100x larger than its predecessor, “GPT-2”, which consists of only 1.5 billion parameters, and 10x larger than Microsoft’s “Turing NLG” language model introduced in 2020, which consists of 17 billion parameters. This suggests greater performance and applicability by GPT-3, which is further corroborated by its ability to outperform “fine-tuned state-of-the-art algorithms” (“SOTA”) spanning other natural language processing (“NLP”) systems, speech recognition and recommendation systems. With 175 billion parameters, GPT-3 can achieve response accuracy of more than 80% in a “few-shots” setting.
Source: “OpenAI Impact Analysis: Microsoft, Google and Nvidia”
As mentioned in the earlier section, the real threat to many existing tech companies today is not ChatGPT, but rather the underlying GPT-3 model itself. The LLM can be applied to verticals beyond just the chatbot:
GPT-3 isn’t programmed to do any specific task. It can perform as a chatbot, a classifier, a summarizer and other tasks because it understands what those tasks look like on a textual level.
Source: Andrew Mayne, Science Communicator at OpenAI
The deployment of GPT-3 across 300 apps “across varying categories and industries, from productivity and education to creativity and games” is a case in point. The LLM has proven to enable “lightning-fast semantic search”, powering a “new genre of interactive stories” in gaming, and generating “useful insights from customer feedback in easy-to-understand summaries” – capabilities far beyond the prompt-and-response function demonstrated via ChatGPT.
But as impressive as GPT-3 is as observed through ChatGPT’s responses spread across the internet in recent weeks, the language model still has limitations that engineers are in the process of trying to fix, including the accuracy of outputs. To be more specific, ChatGPT is actually powered by a refined version of GPT-3, dubbed “GPT-3.5”. And OpenAI is already in the process of working on a next-generation version of the LLM that can be better optimized for multi-vertical deployment and eventual monetization. As previously discussed, “WebGPT” already addresses some of the key limitations of GPT-3 / GPT-3.5 pertaining to accuracy and relevance of responses:
WebGPT has been trained to comb through data available on the internet in real-time to generate more accurate responses, addressing the GPT-3 model’s current limitation of being pre-trained by data dated only up to 2021… WebGPT can also cite sources in its response, addressing concerns over the rate of accuracy in current responses that ChatGPT spits out. Meanwhile, researchers and engineers are still trying to better refine the capability, such that the model could comb through and “cherry-pick” sources that are most reliable and accurate.
Source: “Twilio: Not Yet Profitable, and Already Obsolete”
Google’s Resolve
But Google is not at all behind when it comes to LLMs. In fact, Google is currently one of the leading researchers in the field.
BERT
BERT was developed by Google to enable Search’s ability to better understand queries and prompts today. The LLM is capable of delivering “more useful search results” on Google and underscores how far the online search engine has come since the 2000s when it was already an amazement to see “machine learning correct misspelled Search queries”. BERT is an open-source framework today that has been integrated across a wide array of verticals beyond Google Search that require computers to better understand text prompts, and enable human-like responses. Related functions include “sentiment analysis”, which BERT performs by combing through and understanding digital data such as emails and messages to gauge opinion and emotion.
LaMDA
But Google has done a lot more than just BERT. “LaMDA” (Language Model of Dialogue Applications) is one of them, and has gained significant traction – though not all good – since it was introduced last year. LaMDA is one of most advanced LLMs that Google has been working on. Unlike GPT-3, which is not configured to perform any specific task, LaMDA is “trained on dialogue”:
Language Model of Dialogue Applications”, or “LaMDA”, was also unveiled at this year’s I/O event. LaMDA is trained to engage in conversation and dialogue to help Google better understand the “intent of search queries”. While LaMDA remains in research phase, the ultimate integration of the breakthrough technology into Google Search will not only make the search engine more user-friendly, but also enable search results with greater accuracy.
Source: “Where Will Google Stock Be In 10 Years?”
It is essentially a chatbot-oriented LLM, which has been most commonly linked to discussions on whether it is, or can be, sentient. LaMDA has also been a star figure in recent weeks when it comes to finding a close comparable to ChatGPT. Since LaMDA remains in closed beta testing to only a handful of users, there has been little revealed about its performance (though the recently leaked transcript that sparked debate on whether LaMDA is sentient shows it is pretty smart and capable of understanding text and providing an adequate response). But LaMDA features only 137 billion parameters, a far cry from GPT-3’s 175 billion parameters as discussed in the earlier section. Although the amount of data used to train the LLM is not the sole driver to its performance and accuracy, especially given both GPT-3 and LaMDA are created for different functions, the difference in the number of parameters featured in both does draw greater scrutiny on whether LaMDA is a capable contender to ChatGPT, or GPT-3 in the broader sense. But at least LaMDA proves that Google is not completely out of the loop and far behind in the LLM race, and in fact a key figure in the development of said innovation.
PaLM
In addition to LaMDA, there is also “PaLM” (Pathways Language Model). PaLM is built on Google’s “Pathway” AI architecture, which was introduced in October 2021. Pathway enables a “single model [to] be trained to do thousands, even millions of things”. It is an architecture capable of handling “many tasks at once, [learning] new tasks quickly and [reflecting] a better understanding of the world”. This essentially eliminates the requirement for developing a myriad of new models for learning every modularized individual task. The Pathways infrastructure is also multi-modal, meaning it is capable of processing all of text, images and speech at the same time to generate more accurate responses:
Pathways could enable multimodal models that encompass vision, auditory, and language understanding simultaneously. So whether the model is processing the word “leopard,” the sound of someone saying “leopard,” or a video of a leopard running, the same response is activated internally: the concept of a leopard. The result is a model that’s more insightful and less prone to mistakes and biases.
Source: Google
Now, back to PaLM, the LLM is built on the Pathways AI infrastructure and is a general-purpose model capable of a wide array of language tasks. Essentially, PaLM is a much closer contender to GPT-3 given the wide array of use cases, unlike LaMDA which is trained to be dialogue-specific. It is essentially a “jack of all trades”.
PaLM is also likely capable of greater performance and accuracy when compared to GPT-3. The latest LLM developed by Google features 540 billion parameters, more than 3x larger than GPT-3. While OpenAI’s GPT-3 LLM has proven its ability in outperforming fine-tuned SOTA algorithms with accuracy of more than 80% in a few-shots setting, PaLM can also outperform “the fine-tuned SOTA on a suite of multi-step reasoning tasks, and outperform average human performance on the recently released BIG-bench benchmark”, a standardized test with more than 150 tasks intended to “probe large language models and extrapolate their future capabilities”. PaLM has also proven “discontinuous improvements from model scale” on a wide range of BIG-bench tasks, which suggests that performance will continue to increase steeply as the model scales without significant deceleration:
We also probe emerging and future capabilities of PaLM on the Beyond the Imitation Game Benchmark (BIG-bench), a recently released suite of more than 150 new language modeling tasks, and find that PaLM achieves breakthrough performance. We compare the performance of PaLM to Gopher and Chinchilla, averaged across a common subset of 58 of these tasks. Interestingly, we note that PaLM’s performance as a function of scale follows a log-linear behavior similar to prior models, suggesting that performance improvements from scale have not yet plateaued. PaLM 540B 5-shot also does better than the average performance of people asked to solve the same tasks.
Source: Google
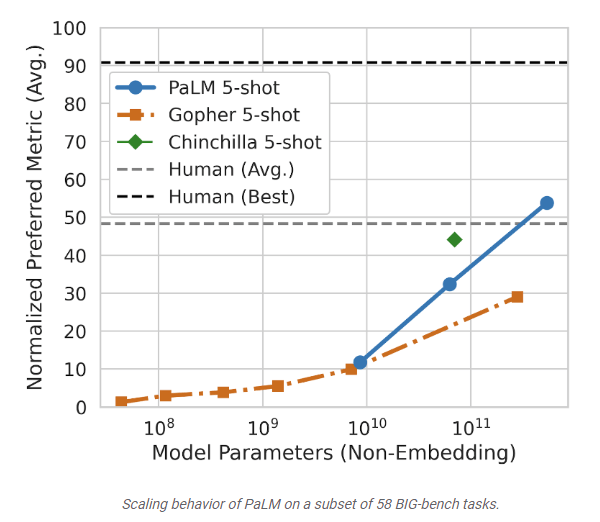
Scaling behaviour of PaLM on a subset of 58 BIG-bench tasks. (Google)
PaLM is also multilingual. Not only is it capable of understanding language tasks in multiple languages like GPT-3 does, it is also trained using a “combination of English and multilingual datasets that include high-quality web documents, books, Wikipedia, conversations and GitHub code” to drive greater accuracy in responses.
Although PaLM’s significant performance capabilities inevitably means a greater computing power requirement, the LLM achieves the highest training efficiency (57.8% hardware floating point operations per second, or FLOPS, utilization) among other models of its scale, underscoring its prowess in not only performance but also efficiency.
Implications for Google
According to founder and CEO of OpenAI, Sam Altman, it costs on average “single-digits cents” per prompt on ChatGPT right now, with potential for further optimization via changes to configuration and also scale of use. For Google, the cost of running each query through Search today is likely significantly lower, given less complexity involved and compute power required by underlying AI models like BERT that currently run the search engine.
The significant volume of queries that run through Google Search everyday likely improves the economies of scale for operating the platform as well. The revenues that Google generates off of ads sold on Google Search today also far exceeds the costs of running the search engine – the company currently boasts gross profit margins of close to 60%, much of which is contributed by its Search advertising business, which also absorbs the significant losses incurred at its Google Cloud Platform (“GCP”) segment.
But continued deployment of capital towards the development of LLMs and other AI investments will continue to be an expensive endeavour for Google. Yet, the company has the ammo to make it happen and turn it into fruition. Its advantages include a significantly robust balance sheet, massive trove of first party search data, and innovative culture:
- Balance sheet strength: AI development is a capital-intensive endeavour, making the continued development of LLMs like LaMDA and PaLM, among other AI capabilities for Search applications and beyond, an expensive undertaking. Yet, the company continues to boast a significant net cash position, with impressive profit margins generated from its existing advertising business. Google Search is not only self-sufficient today, but also capable of generating dollars required to fund growth in adjacent segments like GCP and other investments in Other Bets, inclusive of AI-related R&D. In contrast, OpenAI remains an unprofitable business that requires significant external financing to fund its operations, which subjects it to relatively greater uncertainties pertaining to liquidity means (e.g. exposure to rising borrowing costs, uncertainties over access to funding, etc.).
- First party search data and leading market share advantage: Google Search currently facilities close to 10 billion search requests per day. The prowess is also what makes it one of the most sought-after advertising formats today, despite cyclical headwinds in the industry. The results continue to corroborate the advantage Google has in terms of user reach to protect its market leadership, provided that it continues to keep up with competitive innovations in Search and other AI-enabled technologies. Google’s massive trove of first party search data is also a plus for training and enabling performance of its next-generation LLMs. For perspective, OpenAI’s next-generation WebGPT model is trained to perform live search on data available on the internet via Microsoft’s Bing, implying Google’s LLMs could do the same, or more, via Search.
- Innovative management culture: In addition to running a high-growth and high profitability business, Google also breeds a strong culture of continued innovation. The company understands the importance of innovation to drive sustained growth, profitability, and market leadership. With no immediate signs that the company is looking to plateau and shift from market share expansion to retention, Google sets a strong tone-at-the-top for continued innovation, which will be critical to ensuring the continuation of next-generation LLM developments required to safeguard its market leadership into the future. The qualitative trait effectively sets Google apart from market leaders during the dot-com era that “failed to capitalize on fundamental shifts in computing”, and prevent a “descent into obsolescence” in the rapidly evolving tech industry.
PaLM paves the way for even more capable models by combining the scaling capabilities with novel architectural choices and training schemes, and brings us closer to the Pathways vision: “Enable a single AI system to generalize across thousands or millions of tasks, to understand different types of data, and to do so with remarkable efficiency.”
Source: Google
Final Thoughts
The biggest immediate threat to Google is more so the looming cyclical downturn in ad demand, and broad-based moderation of growth given the sheer size of its sprawling business. This means its longer-term prospects will likely be less lucrative than it once was in the past decade.
Yet, we think the biggest trend after the near-term macro headwinds subside is the shift of focus back onto Google’s innovative and disruptive roots. Google’s continued commitment to innovation – which in our view has garnered the greater attention it deserves in recent weeks since the release of a potential rival product – is what will continue to usher the company through the gradual transition to deeper integration of AI transformer models into services it provides to users worldwide on a daily basis.
And Google has what it takes to make it happen – cash, ambition, and technological capabilities considering the work already in progress. Although market share gains will inevitably moderate, and AI investments will remain capital intensive for a while longer, Google’s balance sheet remains strong with a sustained trajectory of profitable growth into the longer-term, making it a safe, return-generating investment still at current levels.
Disclosure: I/we have a beneficial long position in the shares of GOOG either through stock ownership, options, or other derivatives. I wrote this article myself, and it expresses my own opinions. I am not receiving compensation for it (other than from Seeking Alpha). I have no business relationship with any company whose stock is mentioned in this article.
Thank you for reading my analysis. If you are interested in interacting with me directly in chat, more research content and tools designed for growth investing, and joining a community of like-minded investors, please take a moment to review my Marketplace service Livy Investment Research. Our service’s key offerings include:
- A subscription to our weekly tech and market news recap
- Full access to our portfolio of research coverage and complementary editing-enabled financial models
- A compilation of growth-focused industry primers and peer comps
Feel free to check it out risk-free through the two-week free trial. I hope to see you there!